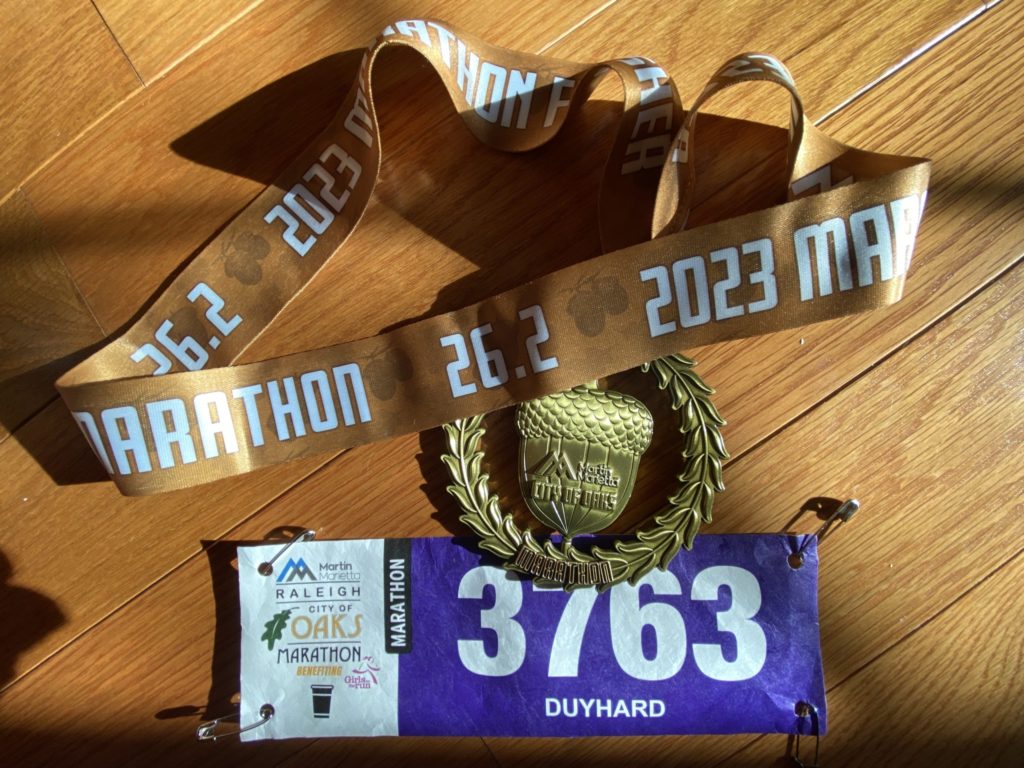
Another marathon is in the books! Yeah, and it’s a sub4 finish. Amidst the verdant backdrop of Raleigh, with the City of Oaks marathon stretching before me, there came a clarity as crisp as the morning air. Every mile under my feet was more than a mere measure of distance; it was a metaphor for the journey in leadership and the resilience required more than ever, in the face of uncertainty nowadays.
The Starting Line: Preparation Meets Opportunity
As the starting gun echoed, it reminded me of the familiar buzz of a new project kickoff that i’ve been experiencing my whole career. Both brim with excitement, a hint of nerves, and the culmination of extensive preparation. Training for a marathon isn’t unlike readying a team for the twists and turns of project development. We set plans, adjust for the environment, and brace for the unforeseen. Yet, in both realms, the first step is always, somewhat, a leap of faith—a belief in the groundwork laid and the strategy set forth.
Finding a Sustainable Pace
The early miles were a celebration, buoyed by the cheers of onlookers and the camaraderie of fellow runners. However, as the course veered away from the crowd, the reality settled in. The marathon, much like the path to leadership, is a personal endeavor. The crowd may cheer you on, but the pavement underfoot asks for your own tenacity, your own steady pace. It’s a delicate dance between pushing the limits and understanding the importance of endurance. In leadership, this translates to balancing ambition with long term sustainability, ensuring your team can maintain their stride without burning out.
The Solitude of the Long-Distance Leader
There was a moment during the race when we marathoners were separated from other distance runners. As the cheerful cacophony of supporters fading away, an enveloping silence took its place. Suddenly, it was just me, the trail, and the long road ahead. It was in this quietude, accompanied only by the rhythmic beating of my footsteps against the morning trail, that I truly felt the parallels between marathon running and the solitude of leadership. In leadership, very often, you venture alone, where the weight of decisions rests heavily on your shoulders. As I continued, the echo of my own breath became a meditation, and each step a conversation with the inner self. I realized the beauty in this inner discourse, learning to draw strength not from the dwindling cheers, but from a deep, intrinsic love, embraced in every stride, every inhalation, every heartbeat.
Every mile of the marathon taught me about resilience, about the drive that comes from within, and about the love for the journey itself. It’s in the quieter moments of leadership when the crowds have dispersed that we learn the most about ourselves. We learn to appreciate the rhythm of our own progress and the internal victories no one else sees.
In leadership, as in marathons, the true reward often lies not in the moment of triumph but in the sum of the steps taken to get there. And as I caught my breath, with the finisher’s medal in hand, I knew this marathon was far more than a physical feat; it was a testament to the journey we all undertake when we choose to lead, to innovate, and to grow.